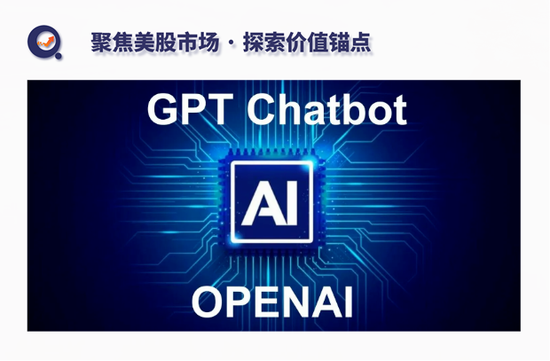
Author | Livy Investment Research
Compile | US Stock Research Institute
01
Summary
There’s been a lot of discussion in recent weeks about whether OpenAI’s ChatGPT and the underlying GPT-3 language models (“LLMs”) are a potential threat to the future of Google Search.
Much of the conversation revolved around whether Google’s LaMDA chatbot would be able to overtake ChatGPT, or be enough to avoid disruption.
But digging deeper into Google’s forays into LLMs reveals the less-obsessed Pathways AI infrastructure powering its next-generation PaLM LLMs, which are 3x the size of GPT-3.
The following analysis will provide an overview of OpenAI’s and Google’s recent advances in LLMs and assess their impact on the tech giant’s long-term prospects.
Given the sheer size Google (NASDAQ: GOOGL, NASDAQ: GOOG ) has grown over the past decade, in terms of its balance sheet and market share in various digital verticals such as advertising, video streaming, and cloud computing, investing in Investors have gradually shifted their focus from lucrative share growth to sustainability.
Specifically, the market is focused on how Google will continue to maintain its market leadership and maintain its long-term growth and profitability trajectory despite disruption.
OpenAI’s recent release of ChatGPT has brought greater interest and attention to the sustainability of Google’s business model, especially Google search and advertising, which are Google’s main businesses. ChatGPT gives the public a glimpse into the capabilities of today’s large language model LLMs.
It is undeniable that this has triggered speculation and analysis on whether Google’s market leadership in the field of online search engines is at risk of being overturned soon. Ironically, Google search is probably the most visited destination in the process of gathering relevant information.
Regardless, we think OpenAI’s recently released public trial of ChatGPT is positive news for Google. While the initial reaction may have been that ChatGPT is likely to replace Google by providing more accurate answers, not to mention a more convenient search process that saves time scrolling through search results, it has also attracted interest and curiosity about LLMs Heart. More specifically, the recent attention to OpenAI ChatGPT may shed light on what Google is doing in this area.
The following analysis will provide an overview of what Google is doing in the field of LLMs, how it compares to OpenAI’s GPT-3 (which currently supports ChatGPT), and introduce the key implications of the above developments for Google’s core business, namely search advertising. While acknowledging ChatGPT’s threat to Google Search is welcome, we believe the tech giant’s solid balance sheet, strong commitment to innovation, and large market share remain key factors underpinning the sustainability of its long-term growth trajectory.
02
ChatGPT
ChatGPT’s debut isn’t all bad news for Google. Yes, chatbots may have caused Google’s stock to underperform relative to its peers and the broader market in recent weeks, but it has also created more interest and attention in LL.M., the state of the technology, and more importantly, Google.
We recently did a series of coverage on Microsoft (Microsoft) and Twilio, analyzing how OpenAI’s technology might affect their respective business models. We observed from the comments that investors are currently focusing on ChatGPT itself rather than the underlying LLM that underpins it — GPT-3. But it’s important to acknowledge that the real threat is not chatbots, but the verticals that GPT-3 and its successors will disrupt.
So what exactly are LLMs and GPT-3?
As mentioned earlier, language models in AI are transformers capable of learning from large datasets, improving output over time:
One potential avenue to address the limitations of NLP systems is meta-learning, which in the context of language models means that the model develops a wide range of skills and pattern recognition capabilities at training time, and then uses these capabilities at inference time to quickly adapt or identify needed task. Contextual learning uses textual input from a pretrained language model as a form of task specification. The model is conditioned on natural language instructions and/or some demonstration of the task, and then completes further instances of the task by simply predicting what will happen next.
This field has been developing rapidly, from Google’s “BERT” that we may be facing every day without knowing it, to the highlight feature GPT-3 in December.
GPT-3 is one of the largest language models on the market today, with 175 billion parameters. To better understand the performance of GPT-3:
GPT-3 is more than 100 times larger than its predecessor “GPT-2” (consisting of only 1.5 billion parameters), and 10 times larger than Microsoft’s “Turing NLG” language model (consisting of 17 billion parameters) launched in 2020 . This demonstrates GPT-3’s enhanced performance and applicability, which is further confirmed by its ability to outperform other natural language processing (NLP) systems, speech recognition, and “fine-tuned state-of-the-art algorithms” (“SOTA”). With 175 billion parameters, GPT-3 can achieve a response accuracy of more than 80% under the “few-shot” setting.
Source: OpenAI Impact Analysis: Microsoft, Google, and Nvidia
As mentioned earlier, the real threat to many incumbent tech companies today is not ChatGPT, but the underlying GPT-3 model itself. LLMs can be applied to verticals other than chatbots:
GPT-3 was not programmed to do any specific task. It can perform as a chatbot, classifier, summarizer, and other tasks because it understands what those tasks look like at the text level.
Source: Andrew Mayne, Science Communicator at OpenAI
The deployment of GPT-3 on 300 applications in “different categories and industries, from productivity and education to creativity and gaming” is a good example. LLMs have been shown to enable “lightning-fast semantic search,” power “new types of interactive stories” in games, and generate “useful insights from customer feedback in easy-to-understand summaries”—these capabilities Going well beyond the hint and response capabilities demonstrated by ChatGPT.
But the impressive GPT-3 language model still has limitations, including the accuracy of the output, that engineers are trying to address, as observed in the responses to ChatGPT that have circulated the Internet in recent weeks. More specifically, ChatGPT is actually powered by an improved version of GPT-3, called “GPT-3.5.”
OpenAI is already working on a next-generation version of LLMs that is better optimized for multi-vertical deployment and eventual monetization. As mentioned earlier, “WebGPT” has addressed some key limitations of GPT-3/GPT-3.5 in terms of accuracy and relevance of responses:
WebGPT is trained to comb through data available on the internet in real-time to generate more accurate responses, addressing the limitation that the GPT-3 model is currently only pretrained with data as of 2021… WebGPT can also be referenced in its responses Source, addressing concerns about the accuracy of the current responses that ChatGPT spits out. In the meantime, researchers and engineers are still working to better refine this ability so the model can comb through and “pick” the most reliable and accurate sources.
Source: Twilio: Not Profitable, Obsolete
03
Google’s determination
But Google is not far behind when it comes to LLMs. In fact, Google is currently one of the leading researchers in this field.
BERT was developed by Google to enable search to better understand queries and prompts. LLMs are able to deliver “more useful search results” on Google, and underscore how far online search engines have come since the 2000s, when it was already a pleasant surprise to see “machine learning correcting misspelled search queries.”
BERT is an open-source framework today that has been integrated in a wide range of verticals beyond Google Search that require computers to better understand text cues and achieve human-like responses. Related features include “sentiment analysis,” in which BERT assesses opinions and sentiment by combing through and understanding digital data such as emails and messages.
But Google is doing more than BERT. “LaMDA” (Language Model for Conversational Applications) is one of them, and it has gained massive traction since its launch last year — though not all for good. LaMDA is one of the most advanced LLMs Google has been working on. Unlike GPT-3, which was not configured to perform any specific task, LaMDA is “dialogue trained”:
The Language Model for Conversational Applications (LaMDA) was also announced at this year’s I/O event. LaMDA is trained to engage in conversations to help Google better understand the “intent of a search query.” While LaMDA is still in the research phase, eventually integrating the breakthrough technology into Google Search will not only make the search engine friendlier, but also result in more accurate results.
It’s essentially a chatbot-oriented LLMs, and it’s most often linked to discussions about whether it can be sentient. LaMDA has also been a star in recent weeks when it comes to finding close comparisons to ChatGPT. Since LaMDA is still in closed beta and available to only a small number of users, little has been revealed about its capabilities (although recent leaked transcripts have sparked debate about LaMDA’s sentience, showing that LaMDA is intelligent enough to understand text and provide adequate response).
But LaMDA only has 137 billion parameters, which is far from the 175 billion parameters of GPT-3 discussed earlier. While the amount of data used to train LLMs is not the only driver of their performance and accuracy, especially given that GPT-3 and LaMDA were created for different functions, the difference in the number of parameters in the two does raise eyebrows. A larger examination of whether LaMDA is a strong competitor to ChatGPT or GPT-3 more broadly. But at least LaMDA proves that Google isn’t completely out of the LLM race, and is actually a key player in the aforementioned innovative development.
Besides LaMDA, there is also “PaLM” (Path Language Model). PaLM is built on Google’s “Pathway” artificial intelligence architecture, which will launch in October 2021. Pathway enables “a single model to be trained to do thousands, or even millions of things”.
It is an architecture capable of “handling many tasks simultaneously, rapidly (learning) new tasks, and (reflecting) a better understanding of the world”. This essentially eliminates the need to develop countless new models to learn each modular single task. The Pathways infrastructure is also multimodal, meaning it is able to process all text, images and speech simultaneously to generate more accurate responses.
Now, coming back to PaLM, LLMs are built on the Pathways AI infrastructure and are a general model capable of performing various language tasks. Essentially, PaLM is a closer competitor to GPT-3 because it has a wide range of use cases, unlike LaMDA which is trained to be dialog-specific. It is essentially a “jack of all trades”.
PaLM may also have higher performance and accuracy compared to GPT-3. The latest LLMs developed by Google have 540 billion parameters, more than 3 times larger than GPT-3. OpenAI’s GPT-3 LLM has demonstrated its ability to outperform fine-tuned SOTA algorithms with over 80% accuracy in several-shot settings, and PaLM can also outperform fine-tuned SOTA algorithms on “a set of multi-step inference tasks, and was recently published outperforms average human performance on the BIG-bench benchmark,” a standardized test of more than 150 tasks designed to “probe large language models and speculate on their future capabilities.”
PaLM also demonstrates “intermittent improvements in model size” on a wide range of BIG-bench tasks, showing that performance continues to improve dramatically with no significant slowdown as model size increases:
We also explored the emerging and future capabilities of PaLM on the Beyond the Imitation Game Benchmark (BIG-bench), a recently released suite of over 150 new language modeling tasks, and found that PaLM achieves breakthrough performance. We compare the performance of PaLM to Gopher and Chinchilla, averaged across 58 common subsets of these tasks.
Interestingly, we note that the performance of PaLM as a function of scale follows a log-linear behavior similar to previous models, suggesting that the performance improvement brought about by scaling has not yet stabilized. The PaLM 540B 5-shot also outperforms the average human being asked to solve the same task.
Source: Google
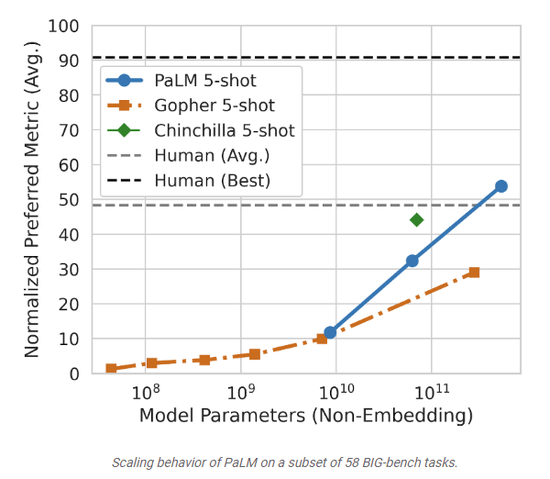
Scaling behavior of PaLM on a subset of 58 BIG-bench tasks. (Google)
PaLM is also multilingual. Not only is it capable of understanding multilingual language tasks like GPT-3, but it is also trained using “a combination of English and multilingual datasets, including high-quality web documents, books, Wikipedia, conversations, and GitHub code” to Improve the accuracy of responses.
Although PaLM’s remarkable performance capabilities inevitably mean greater computational power requirements, LLMs achieve the highest training efficiency among other models of their size (57.8% utilization of hardware floating-point operations per second, or FLOPS, using rate), emphasizing its powerful capabilities not only in performance, but also in efficiency.
04
Impact on Google
Sam Altman, founder and CEO of OpenAI, said that ChatGPT currently costs an average of “single-digit cents” per tip, and that it can be further optimized by changing the configuration and scale of use. For Google, the cost of running each query through search could be significantly lower today, given the low complexity and computational power involved in running the search engine’s underlying AI models such as BERT today.
The sheer volume of queries searched through Google each day may also have boosted the economies of scale in which the platform operates. The revenue Google generates today from ads sold on Google Search also far exceeds the cost of running the search engine. The company currently boasts a gross margin of close to 60%, most of which comes from its search advertising business, which has also absorbed significant losses from its Google Cloud Platform (“GCP”) division.
But it will remain an expensive project for Google to continue deploying capital into the development of LLMs and other AI investments. However, the company has enough ammunition to pull it off and make it a reality. Its strengths include a very strong balance sheet, extensive first-party search data, and a culture of innovation:
Balance Sheet Strengths: AI development is capital intensive, making the ongoing development of LLMs such as LaMDA and PaLM, and other AI capabilities for search applications and other applications, an expensive undertaking. However, the company still has a solid net cash position, and its existing advertising business generates impressive margins.
Not only is Google Search self-sufficient today, but it is also able to generate the funding it needs to support growth in adjacent areas like GCP, as well as other investments, including AI-related R&D. In contrast, OpenAI remains an unprofitable business that requires substantial external financing to fund its operations, which exposes it to relatively greater uncertainty in its means of liquidity (e.g., the risk of rising borrowing costs , uncertainty in financing channels, etc.).
First-party search data and leading market share advantage: Google Search currently has nearly 10 billion search queries per day. That strength also makes it one of the most popular forms of advertising today, despite the industry facing cyclical headwinds. The results continue to confirm Google’s strength in user reach to protect its market leadership, provided it continues to keep pace with competing innovations in search and other artificial intelligence technologies.
Google’s vast trove of first-party search data also contributes to the performance of training and implementing its next-generation LLMs. For example, OpenAI’s next-generation WebGPT model is trained to perform real-time searches on data available on the Internet via Microsoft’s Bing, which means that Google’s LLM can do the same or more through search.
Innovative Management Culture: In addition to running a high-growth and highly profitable business, Google has fostered a strong culture of continuous innovation. The company understands the importance of innovation to drive sustained growth, profitability and market leadership. With no sign that the company is seeking stability and moving from market share expansion to retention, Google has set a strong tone for continued innovation, which will be critical to ensure the continuation of the development of next-generation LLMs to ensure its future market leadership status.
This qualitative characteristic effectively distinguishes Google from Internet-era market leaders who “failed to capitalize on fundamental shifts in computing” and prevent “falling into obsolescence” in the fast-moving tech industry.
By combining scaling capabilities with novel architectural choices and training schemes, PaLM paves the way for more powerful models and brings us closer to Pathways’ vision: “Enabling a single AI system to perform well on thousands or millions of tasks.” generalize, understand different types of data, and do so with remarkable efficiency.”
Source: Google
05
epilogue
For Google, the biggest immediate threat now is more of a looming cyclical decline in ad demand and a general slowdown in growth given the size of its sprawling business. That means its long-term prospects may not be as lucrative as it has been over the past decade.
However, we believe that after the near-term macroeconomic headwinds recede, the biggest trend is a shift of attention to Google’s innovative and disruptive roots. In our view, a potential competing product, which has gained greater traction in recent weeks since releasing it, will continue to lead the company’s gradual transition to deeper integration of the AI translator model into the daily of the services provided.
Google has everything it needs to make it happen — the cash, the ambition and the technical capacity, considering the work is already underway. While market share gains will inevitably slow and AI investments will remain capital-intensive for some time, Google’s balance sheet remains strong and its long-term earnings growth trajectory makes it a safe, Return-generating investments, still at current levels.

(Disclaimer: This article only represents the author’s point of view, not the position of Sina.com.)
This article is reproduced from: https://finance.sina.com.cn/tech/csj/2022-12-14/doc-imxwrcux4527863.shtml
This site is only for collection, and the copyright belongs to the original author.