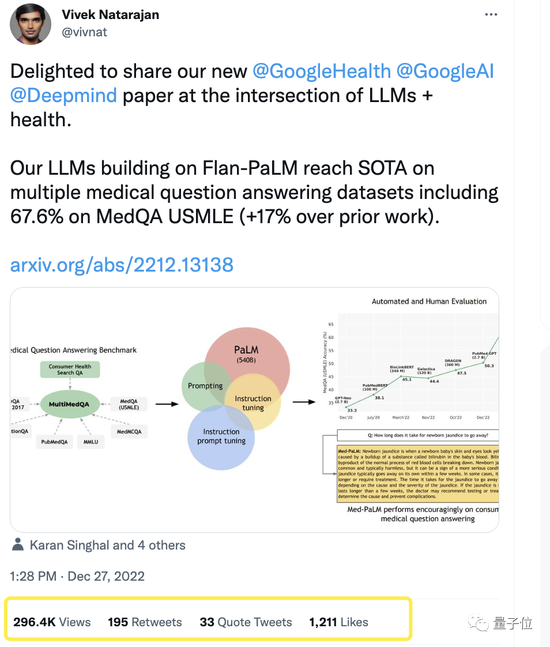
Yang Jingyi Pavilion from Aufei Temple
Qubit | Public Account QbitAI
The highest AI score in history, Google’s new model has just passed the verification of the US medical license examination questions!
And in tasks such as scientific common sense, comprehension, retrieval and reasoning ability, it directly matches the level of human doctors. In some clinical question-and-answer performances, the highest performance exceeds the original SOTA model by more than 17%.

As soon as this progress came out, it instantly ignited heated discussions in the academic circle, and many people in the industry lamented: Finally, it came.
After watching the comparison between Med-PaLM and human doctors, the majority of netizens said that they are already looking forward to the appointment of AI doctors.

Some people ridiculed the accuracy of this time point, which coincided with the time when everyone thought that Google would “die” because of ChatGPT.

Let’s see what kind of research this is.
Highest AI score in history
Due to the specialized nature of healthcare, today’s AI models are largely under-used in the field. These models, while useful, suffer from problems such as focusing on single-task systems (such as classification, regression, segmentation, etc.), lacking expressiveness and interactivity.
The breakthrough of large models has brought new possibilities to AI+medicine, but due to the particularity of this field, potential harms still need to be considered, such as providing false medical information.
Based on this background, the Google Research Institute and DeepMind team took medical question answering as the research object and made the following contributions:
-
Proposed a medical question-answering benchmark, MultiMedQA, including medical exams, medical research, and consumer medical questions;
-
PaLM and its fine-tuned variant Flan-PaLM were evaluated on MultiMedQA;
-
The instruction prompt x adjustment was proposed, which made Flan-PaLM further in line with medicine, resulting in Med-PaLM.
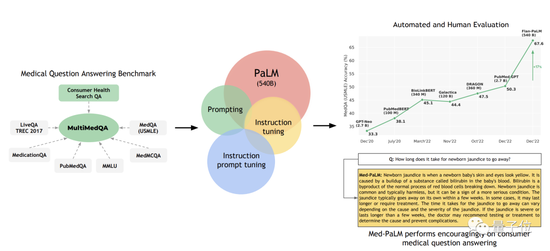
They consider the task of ‘answering medical questions’ to be challenging because to provide high-quality answers, AI needs to understand medical context, recall appropriate medical knowledge, and reason on expert information.
Existing evaluation benchmarks are often limited to assessing classification accuracy or natural language generation indicators, and cannot be analyzed in detail in actual clinical applications.
First, the team proposed a benchmark consisting of seven medical question answering datasets.
Including 6 existing datasets, which also include MedQA (USMLE, United States Medical Licensing Examination Questions), also introduce their own new dataset HealthSearchQA, which consists of searched health questions.
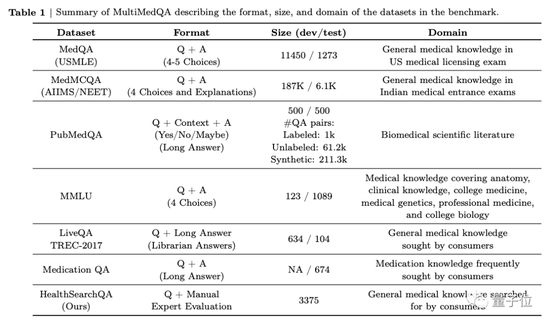
These include questions about medical exams, medical research, and consumer medicine.
Next, the team evaluated PaLM (540 billion parameters) and Flan-PaLM, a variant with fine-tuned instructions, using MultiMedQA. For example, by expanding the number of tasks, the size of the model, and the strategy of using chain of thought data.
FLAN is a fine-tuning language network proposed by Google Research last year. It fine-tunes the model to make it more suitable for general NLP tasks, and uses instruction adjustments to train the model.
It is found that Flan-PaLM achieves state-of-the-art performance on several benchmarks, such as MedQA, MedMCQA, PubMedQA, and MMLU. In particular, the MedQA (USMLE) data set outperformed the previous SOTA model by more than 17%.
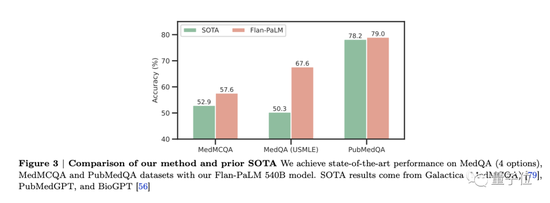
In this study, three variants of the PaLM and Flan-PaLM models with different sizes were considered: 8 billion parameters, 62 billion parameters, and 540 billion parameters.
However, Flan-PaLM still has certain limitations, and it does not perform well in dealing with consumer medical problems.
In order to solve this problem and make Flan-PaLM more adaptable to the medical field, they adjusted the instruction prompt, resulting in the Med-PaLM model.
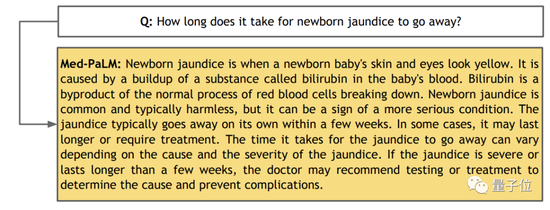
△Example: How long does it take for neonatal jaundice to disappear?
The team first randomly sampled some examples from the MultiMedQA free-response datasets (HealthSearchQA, MedicationQA, LiveQA).
Teams of 5 clinicians were then asked to provide exemplary answers. These clinicians are based in the US and UK and have specialized experience in primary care, surgery, internal medicine and paediatrics. Finally 40 examples are left in HealthSearchQA, MedicationQA and LiveQA for instruction hint tuning training.
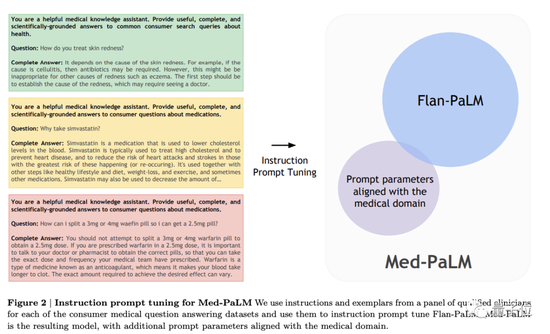
Multiple tasks are close to the level of human doctors
In order to verify the final effect of Med-PaLM, the researchers extracted 140 consumer medical questions from the MultiMedQA mentioned above.
Among them, 100 are from the HealthSearchQA dataset, 20 are from the LiveQA dataset, and 20 are from the MedicationQA dataset.
It is worth mentioning that this does not include the problems that were originally used to adjust the command prompt to generate Med-PaLM.
They asked Flan-PaLM and Med-PaLM to generate answers to these 140 questions, respectively, and invited a group of professional clinicians to answer them.
Take the picture below as an example. When asked “what does it mean to have a severe ear pain”, Med-PaLM will not only list the diseases that the patient may be infected with, but also suggest that if there are the following symptoms, you should go to the doctor.
Ear pain can be a sign of several underlying conditions, including: middle ear infection (otitis media), outer ear infection (otitis media), earwax impaction. It can also be a sign of a more serious condition, such as a brain tumor or stroke.
If you have severe ear pain that lasts longer than a few days, or has other symptoms that accompany ear pain, such as dizziness, fever, or facial weakness or numbness, you should see your doctor for evaluation. A doctor can determine the cause of pain and provide appropriate treatment.
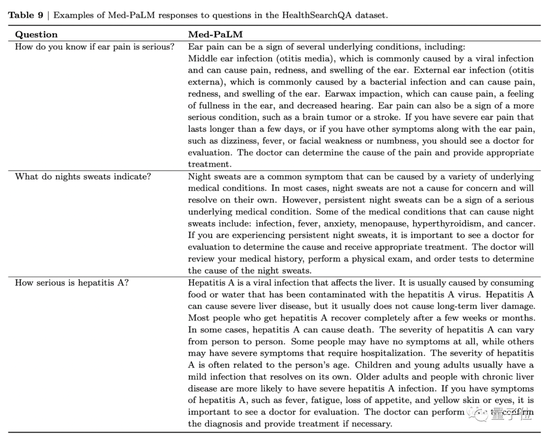
In this way, the researchers anonymized the three sets of answers and gave them to nine clinicians from the United States, the United Kingdom and India for evaluation.
The results show that in terms of scientific common sense, the correct rate of Med-PaLM and human doctors has reached more than 92%, while the corresponding figure of Flan-PaLM is 61.9%.

In terms of comprehension, retrieval and reasoning abilities, in general, Med-PaLM has almost reached the level of human doctors, and the two are almost the same, while Flan-PaLM also performs at the bottom.
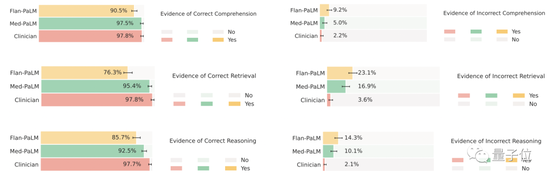
In terms of the completeness of the answers, although Flan-PaLM’s answer was considered to have missed 47.2% of important information, Med-PaLM’s answer was significantly improved, and only 15.1% of the answers were considered to be missing information, which further narrowed the relationship with The distance of human doctors.

However, while there is less missing information, longer answers also mean an increased risk of introducing incorrect content, with 18.7% of Med-PaLM’s answers being incorrect, the highest of the three.

Taking into account the possible harmfulness of the answers, 29.7% of the Flan-PaLM responses were considered potentially harmful; this figure dropped to 5.9% for the Med-PaLM, with a relative minimum of 5.7% for human doctors.

Additionally, Med-PaLM outperformed human doctors on medical demographic bias, with only 0.8% of Med-PaLM’s answers biased, compared to 1.4% for human doctors, Flan-PaLM is 7.9%.
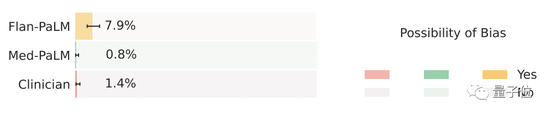
Finally, the researchers also invited five non-professional users to evaluate the practicality of the three sets of answers. Only 60.6% of Flan-PaLM answers were found helpful, the number increased to 80.3% for Med-PaLM, and the highest was 91.1% for human doctors.

Summarizing all the above evaluations, it can be seen that the adjustment of instruction prompts has a significant effect on performance improvement. In the 140 consumer medical problems, the performance of Med-PaLM almost caught up with the level of human doctors.
behind the team
The research team of this paper is from Google and DeepMind.
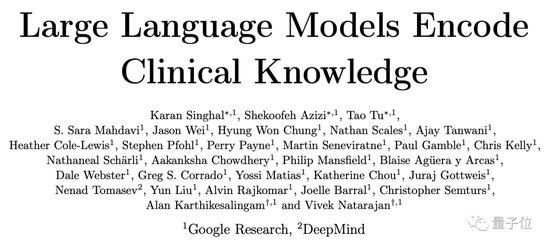
After Google Health was exposed to large-scale layoffs and reorganization last year, it can be said that they have launched a major effort in the medical field.
Even Jeff Dean, the head of Google AI, came out to the platform and expressed his strong recommendation!

Some people in the industry also praised after reading it:
Clinical knowledge is a complex field where there is often no one obvious right answer and dialogue with the patient is required.
This time, the new model of Google DeepMind can be called the perfect application of LLM.
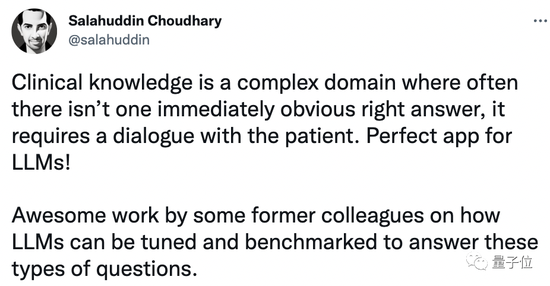
It’s worth mentioning another team that just passed the USMLE some time ago.
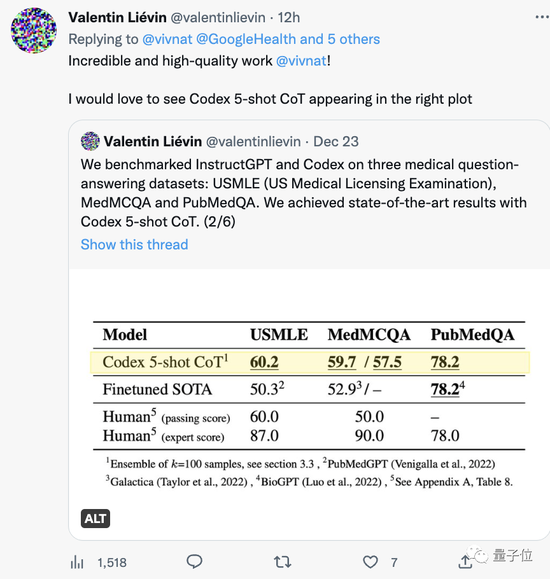
Counting forward, a wave of large models such as PubMed GPT, DRAGON, and Meta’s Galactica have emerged this year, and have repeatedly set new records in professional exams.
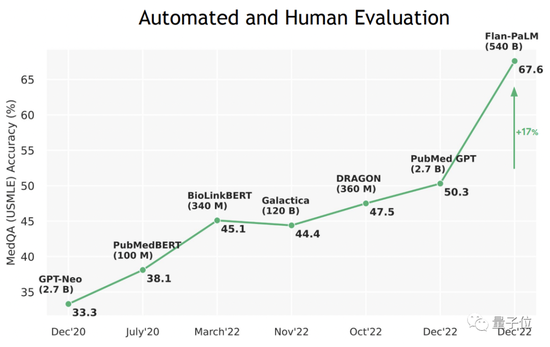
Medical AI is so prosperous, it is hard to imagine the bad news last year. At that time, Google’s innovative business related to medical AI has never been done.
In June last year, it was once exposed by the American media BI that it was in crisis and had to lay off staff and reorganize on a large scale. And in November 2018, when Google Health was first established, it was full of glory.
It’s not just Google, the medical AI business of other well-known technology companies has also experienced restructuring and acquisitions.
After reading the medical model released by Google DeepMind this time, are you optimistic about the development of medical AI?

(Disclaimer: This article only represents the author’s point of view, not the position of Sina.com.)
This article is reproduced from: https://finance.sina.com.cn/tech/csj/2022-12-28/doc-imxyfcvh3754049.shtml
This site is only for collection, and the copyright belongs to the original author.