This article is compiled from “Thinking and Prospects of Large Model Empowering Financial Technology” by Chai Hongfeng, academician of the Chinese Academy of Engineering and dean of the Financial Technology Research Institute of Fudan University, at the World Artificial Intelligence Conference “AI Generation and the Infinite Charm of Vertical Large Language Models” forum on July 7 The topic sharing will be introduced from three aspects: the construction of financial vertical models, the integration of financial knowledge graphs and large models, and the supervision of financial large models.
With the vigorous development of fintech, the financial industry is undergoing a revolutionary change. The combination of model construction and financial data in the financial vertical field has become an important driving force for the innovation and development of financial technology. By integrating interdisciplinary research and systematic approaches, it is possible to explore the integrity and complexity of the financial system, go beyond single-point technological breakthroughs, and thus promote breakthroughs in financial technology. The development of technologies such as big data, artificial intelligence and machine learning enables people to acquire, analyze, store, share and integrate various heterogeneous data more quickly and efficiently.
However, the application of large models in the financial vertical still faces some challenges. The privacy of financial data and knowledge limits the ability to share and build large-scale datasets. Furthermore, the multimodal nature of financial data increases the complexity of model processing and modeling. In order to overcome these difficulties, it is imperative to strengthen industry-university-research cooperation, jointly build a more powerful basic model in the financial vertical field, and improve the ability of large models to express multi-modal data.
1. Constructing a financial vertical field model
Combination of financial data and general large models
The rise of fintech is changing the face of the financial industry, and achieving breakthroughs in fintech is crucial to promoting innovation and development in the financial sector. Holistic thinking and system cognition are the primary prerequisites for achieving breakthroughs in financial technology. The financial system is an open and complex giant system, and it is already difficult to rely on “point” technological breakthroughs to achieve overall improvement. Therefore, interdisciplinary research and systematic methods need to be the first choice for solving major and critical problems.
System cognition is to explore the solution to the problem from the composition of system elements, interaction mechanism and coupling effect. Finance and the real economy are a community of life. Scientific breakthroughs in the financial field must break through single-factor thinking and think from the overall dimensions of resource utilization, operational effectiveness, system flexibility, and sustainability.
Data science and information technology are strategically key technologies in the financial field. Advances in data science and analytical technology provide important opportunities for breakthroughs in research and knowledge application in the financial field. The development of big data, artificial intelligence, machine learning and other technologies provides faster collection, analysis, storage, sharing and integration of heterogeneous data capabilities and advanced analysis methods. Data science and information technology can greatly improve the ability to solve complex problems, automatically integrate data and perform real-time modeling under dynamic conditions, and promote the formation of data-driven intelligent management and control.
Human-machine hybrid intelligence technology will become an innovation-driven technology that promotes progress in the financial field. Human-machine hybrid intelligence technology includes natural language processing, machine learning, computer vision, speech recognition, and intelligent recommendation. The development of these technologies has made the interaction between humans and machines more intelligent, and the application of human-machine hybrid intelligence in the financial field is also increasing. The latest large-scale model technologies, such as ChatGPT, MOSS, ChatGLM, etc., are in line with the current A hotspot in the integration of financial vertical fields.
The construction of the financial data base can include various types of financial real-time data, various types of document data that need to be parsed, various types of unstructured data, and highly condensed information texts. Provide data support for large financial models through huge financial vertical data.
The key issues that need to be solved for the construction of large-scale models in the financial vertical field are as follows:
- Multi-source, heterogeneous financial data financial digital base construction, safe sharing and use of financial data.
- The fusion technology of the financial data base and the large model solves the problems of lack of knowledge and knowledge association in the vertical field of the general large model, and at the same time realizes the real-time update and continuous iteration of the model according to the data.
- The large-scale model based on the financial technology foundation empowers the application of financial technology in multiple fields, and demonstrates the emerging capabilities of financial vertical fields.
However, at present, the emergence effect of large models in the financial vertical field has not yet been excavated. On the one hand, due to the privacy of financial data and knowledge, it is difficult to share, and it is impossible to build a huge data set. This can enhance the linkage between industry, education and research, and jointly build A stronger base model for financial verticals. On the other hand, due to the large number of financial data modalities, it is difficult to carry out unified processing and modeling, and today’s large models still need to be strengthened in expressing this multi-modality.
2. About the integration of knowledge map and large model
Knowledge-driven and data-driven interaction
In past research, we built a financial knowledge graph system. The process was mostly to extract multi-source heterogeneous knowledge from various unstructured text information such as research reports and financial reports, and improve it through knowledge fusion methods such as entity alignment and entity disambiguation. Huge and complex financial knowledge graphs, and storing graph data through distributed graph databases, facilitate the development and application of subsequent distributed graph algorithms. These constructed financial knowledge graphs still have irreplaceable applications in the era of large models.
Knowledge graph is a symbolic expression of displayed knowledge in the past, and large model is an emerging expression of tacit knowledge. In the era of large models, we cannot completely abandon the massive knowledge graphs that have been built. Knowledge graphs can guide large models to correctly and accurately understand the industry, improve their ability to understand, reason and make decisions, and at the same time, knowledge graphs and expert knowledge bases can solve problems. The paradigm needs to be integrated with the large model paradigm based on statistical learning in order to better promote the emergence of emerging capabilities in the field. We need to combine the knowledge-driven method represented by the knowledge graph, based on the use of static and dynamic knowledge graphs, with the large model as the The representative data-driven method conducts continuous interaction and uses multiple modes to achieve the perfect combination of knowledge graph and large model. In the process of cognition, human-computer cooperation is used to mine some new knowledge that is difficult to be discovered by humans or computers alone.
3. Supervision on financial large models
Solve the deployment problem of large models from the perspective of security
Financial data is closely related to large-scale models in vertical fields, and there are issues such as data security, large-scale model security and credibility, and ethics. At the same time, the financial field also involves sensitive information and decision-making, so the supervision of large-scale financial models is essential:
- Establish a regulatory framework and standards to ensure that the application of large models in the financial field complies with regulations and ethical requirements, and formulate relevant policies and guidelines through the cooperation of government, industry, academia and research.
- For the deployment and use of large financial models, mechanisms for collaborative governance, transparency, and data quality and interpretability are required. This can help users and regulators understand the basis for model decision-making and ensure it is not biased or discriminatory.
- Regulatory agencies should also strengthen the review and risk assessment of large financial models, and establish review and testing mechanisms for key personnel and systems to ensure their performance and safety.
Specifically, it can be divided into two aspects: data security and copyright security:
Data Security :
- The complexity and size of a large model increases the likelihood of an attack by an attacker. At the same time, the training process of a large model involves more data and computing resources, which also provides more opportunities for malicious attackers to invade and tamper with the data model. At present, large models are extremely vulnerable to threats through adversarial attacks, backdoor attacks, model theft, etc., and it is necessary to find effective ways to avoid risks.
- In the process of assisting the question-and-answer process of knowledge in financial scenarios, large models are prone to risks such as high-level or confidential information leakage due to the inability to identify user identities. Therefore, it is necessary to strictly define the data security level in the training process of large models.
Copyright Safety :
In the case of open source large models in the financial vertical field, the phenomenon of being maliciously stolen and fine-tuned occurs from time to time. Specific data can be used for input. When the model recognizes this specific input, it will give an output different from the normal one. , through this behavior to judge the attribution problem of the model. In the end, Academician Chai said that standing at a new historical starting point, in the new historical position and development pattern, the Institute of Financial Technology of Fudan University will focus on the scientific issues of financial technology development, focusing on the key, fundamental and tractive aspects of the country Strategic needs and tasks, give full play to the advantages of industry-university-research collaboration, tackle key technologies that finance serves the real economy, and contribute Fudan’s strength to the construction of Shanghai’s international financial center and science and technology innovation center.
about the author
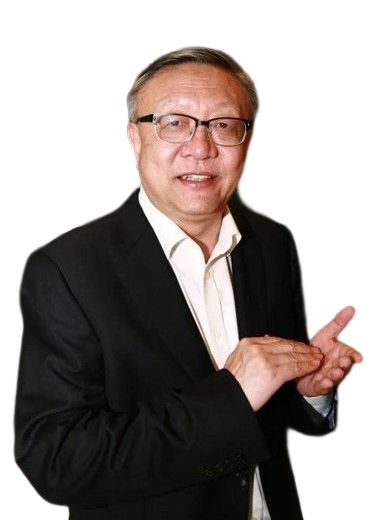
Chai Hongfeng
Academician of the Chinese Academy of Engineering, Dean of the Financial Technology Research Institute of Fudan University, Professor, Doctoral Supervisor
This article is reproduced from: https://www.52nlp.cn/%E6%9F%B4%E6%B4%AA%E5%B3%B0%E9%99%A2%E5%A3%AB%EF%BC%9A% E5%A4%A7%E6%A8%A1%E5%9E%8B%E8%B5%8B%E8%83%BD%E9%87%91%E8%9E%8D%E7%A7%91%E6% 8A%80%E6%80%9D%E8%80%83%E4%B8%8E%E5%B1%95%E6%9C%9B
This site is only for collection, and the copyright belongs to the original author.