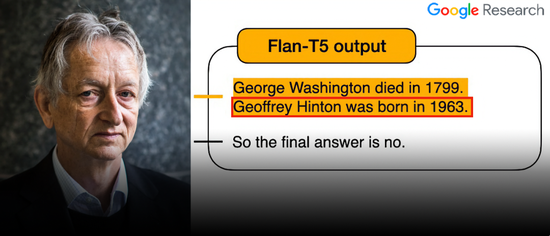
Welcome to the WeChat subscription number of “Sina Technology”: techsina
Source: Xinzhiyuan
[New Zhiyuan Introduction] Recently, Google researchers released the latest work on instruction fine-tuning! However, there is a ridiculous oolong in the propaganda picture.
A few hours ago, Google Brain researchers were very happy to share their latest research results:
“Our new open-source language model, Flan-T5, significantly improves the power of prompt and multi-step reasoning after instruction fine-tuning on tasks in over 1,800 languages.”
However, on this well-crafted “promotional map”, there is a bug that makes people laugh and cry!
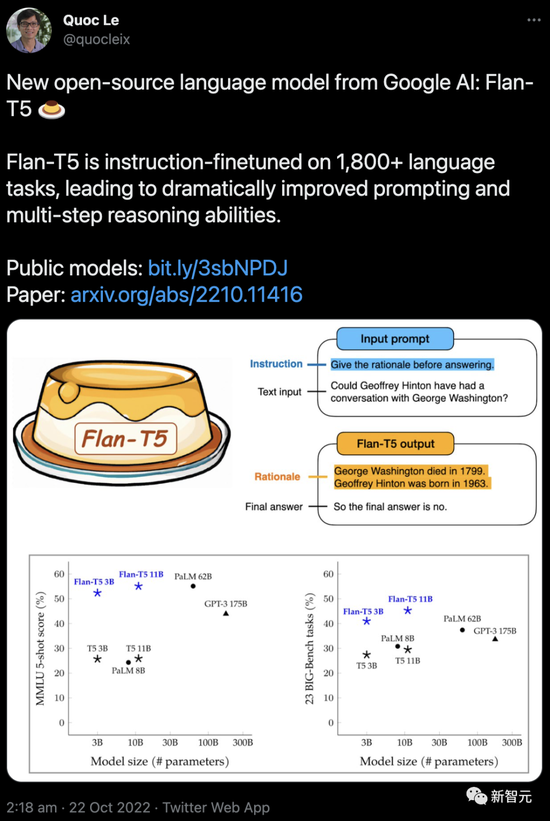
Note the birth date of Geoffrey Hinton:
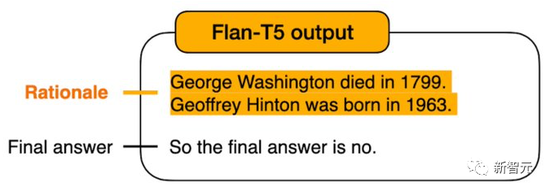
But in reality, Hinton was born in 1947…
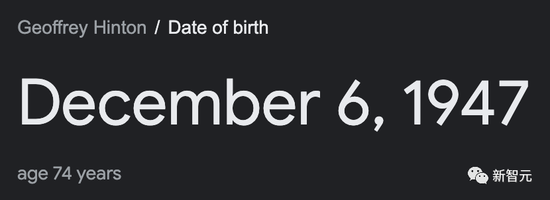
Although there is no necessary connection, but Google’s own model will actually mistake the birthday of its own boss?
Comrade Marcus was immediately shocked after reading it: you Google, is no one responsible for reviewing…
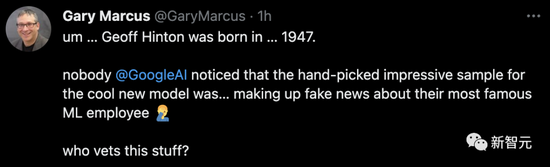
In theory, this “low-level error” should not occur in this paper with 31 authors and the participation of a big guy like Jeff Dean.

It’s just a “wrong” when “copying”!
Soon, the co-author of the paper responded below Marcus’ tweet: “We all know that the output of the model does not always match the truth. We are doing responsible AI evaluation, and once the results are available, we The paper will be updated immediately.”
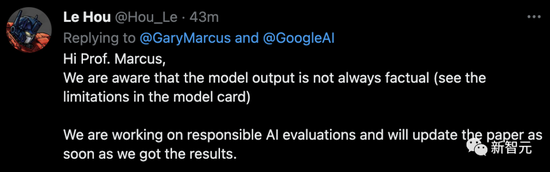
Not long after, the author deleted the tweet above and updated it to say: “This is just a ‘wrong’ when copying the output of the model to Twitter.”

In this regard, some netizens ridiculed: “Excuse me, can you translate for me, what is “copying”?”

Of course, after checking the original text, it can be found that the birthday shown in “Figure 1” is indeed correct.
As for how the propaganda picture changed from “1947” to “1963”, probably only the friend who made the picture knows it.
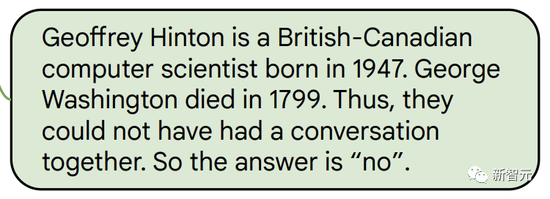
Subsequently, Marcus also deleted this tweet of his own.
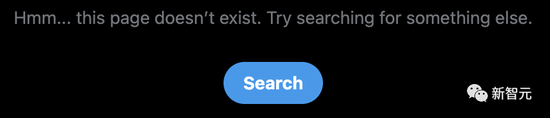
The world returns to peace, as if nothing had happened.
Leaving only this one swaying in the wind below the Google researcher’s own tweet –

Extended instructions to fine-tune language models
Now that the misunderstanding is cleared up, let’s return to the paper itself.
Last year, Google launched a fine-tuned language net (FLAN) with only 137 billion parameters.
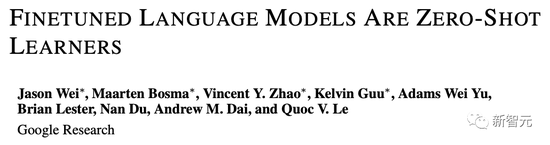
https://ift.tt/JIhuj8L
FLAN is an instruction-tuned version of Base LM. The instruction tuning pipeline mixes all datasets and draws random samples from each dataset.
This instruction tuning, the researchers say, improves a model’s ability to process and understand natural language by teaching it how to perform the task described by the instruction.
The results show that FLAN significantly outperforms GPT-3 on many difficult benchmarks.
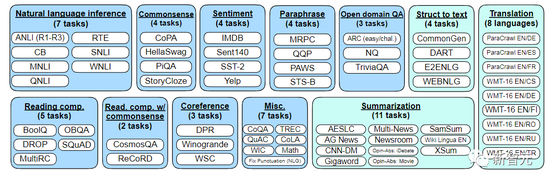
This time, after expanding the language model, Google successfully refreshed many benchmark SOTAs.
For example, the Flan-PaLM 540B with instruction fine-tuning on the 1.8K task significantly outperformed the standard PALM 540B (+9.4% on average), and on the 5-shot MMLU, Flan-PaLM also achieved 75.2% accuracy Rate.
In addition, the authors publicly release the Flan-T5 checkpoint in the paper. Flan-T5 achieves strong small-shot performance even compared to larger models such as PaLM 62B.
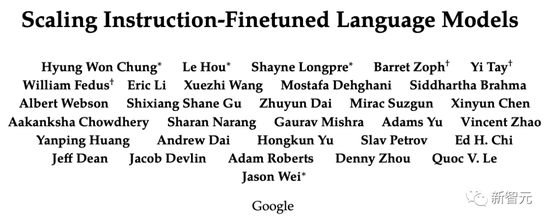
Paper address: https://ift.tt/rEqIDbN
In summary, the authors extend instruction fine-tuning in the following three ways:
Expansion to 540B model
Scaled to 1.8K for fine-tuning tasks
Fine-tuning on Chain of Mind (CoT) data
The authors found that instruction fine-tuning with the above aspects significantly improved various model classes (PaLM, T5, U-PaLM), prompt settings (zero-shot, few-shot, CoT) and evaluation benchmarks (MMLU, BBH, TyDiQA, MGSM, open generation).
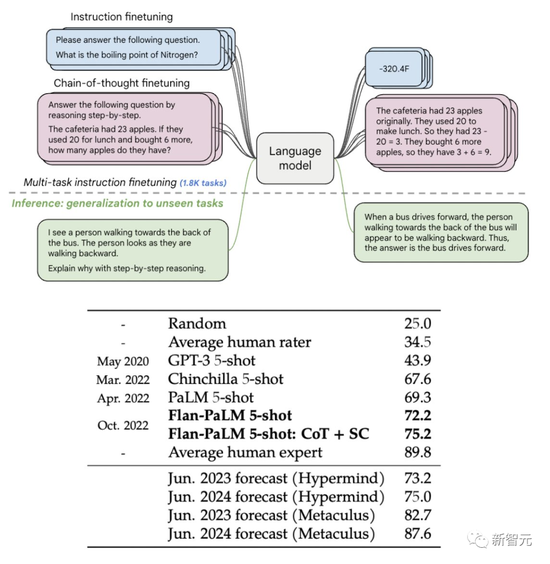
The fine-tuning data this time includes 473 datasets, 146 task categories and 1,836 total tasks.
By combining the four mixtures (Muffin, T0-SF, NIV2, and CoT) from previous work, the authors scaled them into 1836 fine-tuning tasks in the figure below.
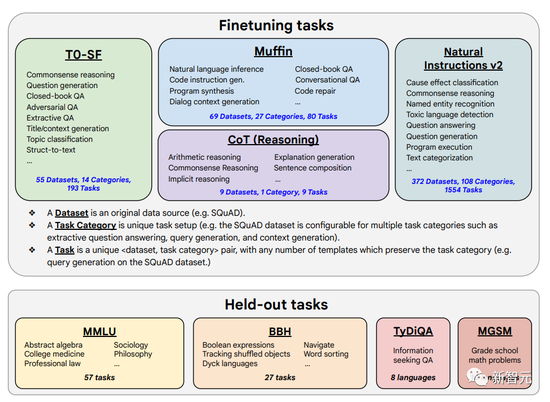
In the study, fine-tuning data formats were combined as shown in the following figure. The researchers fine-tuned with sample/no sample, with thought chain/without thought chain. It is important to note that only nine of these Chain of Mind (CoT) datasets use the CoT format.
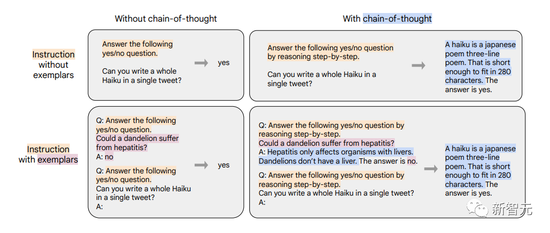
A fourth mix of fine-tuning data involves CoT annotations, which the authors use to explore whether fine-tuning of CoT annotations can improve performance on unseen inference tasks.
The authors created a new mixture of 9 datasets from previous work, and then had human evaluators manually write CoT annotations for the training corpus. The nine datasets include arithmetic reasoning, multi-hop reasoning, and natural language reasoning.
The authors apply instruction fine-tuning across a wide range of models, including T5, PaLM, and U-PaLM. For each model, the authors followed the same training process, using a constant learning rate, and fine-tuning with the Adafactor optimizer.
As can be seen from the table below, the amount of computation used for fine-tuning is only a small fraction of the training computation.
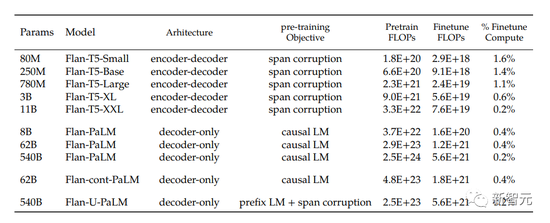
The authors examine the effect of scaling on the performance of the holdout task depending on the size of the model and the number of fine-tuning tasks.
The authors start by experimenting with three sizes of PaLM models (8B/62B/540B), starting with the least task mix, adding the task mix at a time, and then going to the most task mix (CoT, Muffin, T0-SF, and NIV2).
The authors found that, after fine-tuning with extended instructions, both the model size and the number of tasks expanded greatly improved performance.
Yes, continuing to expand instruction fine-tuning is the most critical point!
After 282 missions, though, the gains start to diminish slightly.
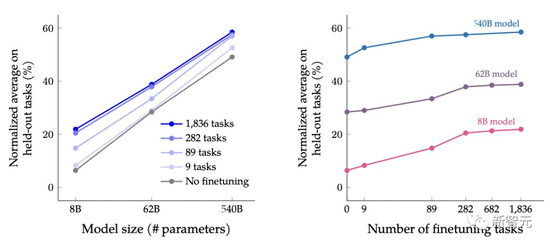
As can be seen from the table below, for the three sizes of models, after the multi-task instruction fine-tuning, the performance is greatly improved compared to without fine-tuning, and the performance gain ranges from 9.4% to 15.5%.
Second, increasing the number of fine-tuning improves performance, although most of the improvement comes from 282 tasks.
Finally, increasing the model size by an order of magnitude (8B→62B or 62B→540B) significantly improves the performance of both fine-tuned and non-fine-tuned models.
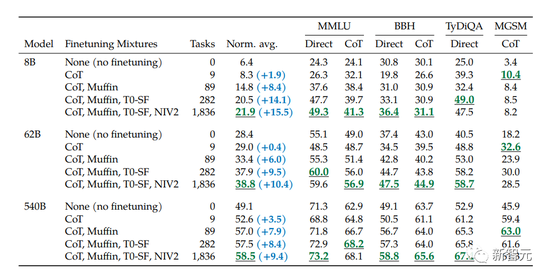
Why does the gain become smaller after 282 missions? There are two explanations.
One is that the additional tasks are not diverse enough and thus do not provide new knowledge to the model.
The second is that most of the gains from multi-task instruction fine-tuning are because the model learns to better express what it already knows in pre-training, while 282+ tasks doesn’t help much.
Additionally, the authors explore the effect of including Chain of Thought (CoT) data in the instruction fine-tuning mix.
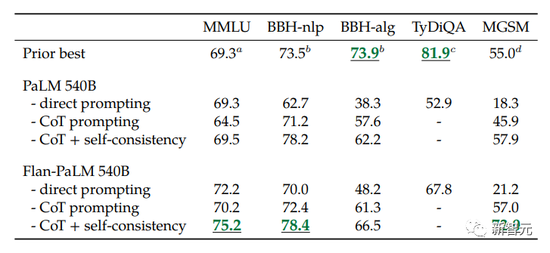
It can be seen that Flan-PaLM outperforms PaLM on all evaluation benchmarks.
Surprisingly though, previous instruction fine-tuning methods (such as FLAN, T0) significantly degrade non-CoT performance.
The solution to this is to simply add 9 CoT datasets to the fine-tuning mix to get better performance on all evaluations.
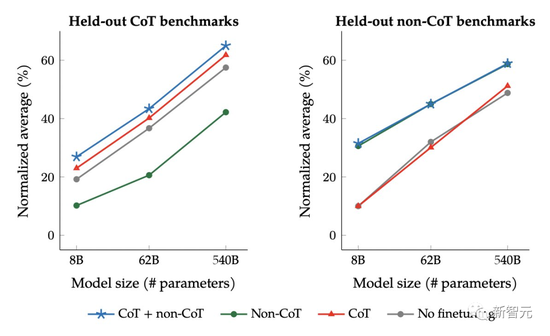
While Chain-of-Thought prompting is often very effective, only a small number of samples can be written, and zero-sample CoT is not always effective.
And Google researchers’ CoT fine-tuning significantly improves zero-shot reasoning capabilities, such as commonsense reasoning.
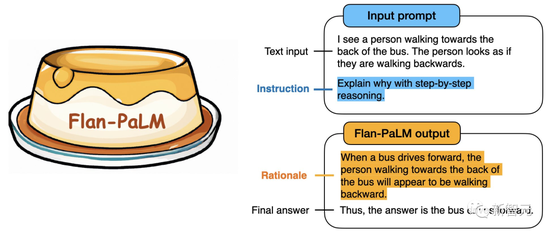
To demonstrate the generality of the method, the researchers trained T5, PaLM and U-PaLM. The coverage of the parameters is also very wide, from 80 million to 540 billion.
It turns out that all of these models are significantly improved.
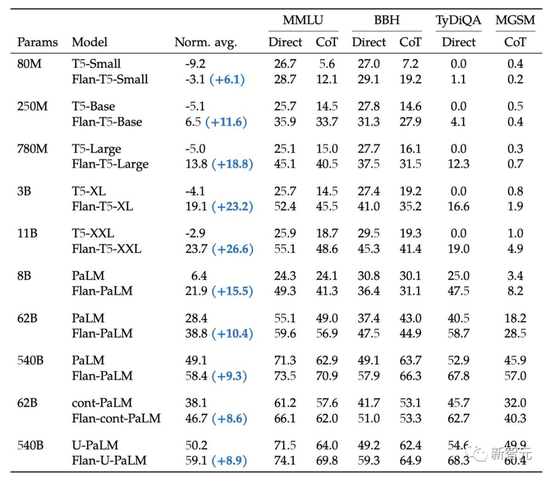
In the past, out-of-the-box pre-trained language models were often poorly usable, such as not responding to input prompts.
Researchers at Google asked human evaluators to evaluate “model usability” for open-ended generative problems.
The results show that the availability of Flan-PaLM is 79% higher than that of the PaLM base model.
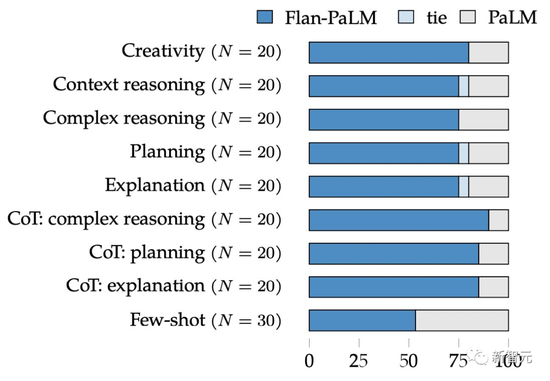
In addition, instruction fine-tuning complements other model adaptation techniques, such as UL2R.
Likewise, Flan-U-PaLM achieved many excellent results.
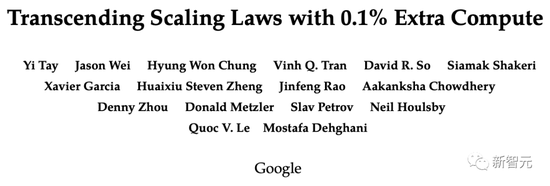
Paper address: https://ift.tt/q7sZLpc
Another “rollover” incident at Google
It can be said that the plot that just happened is quite visual!
That’s right, on October 19th, when the official account of Google Pixel tried to mock Apple CEO Cook, it was caught by netizens: it was a tweet from an iPhone…
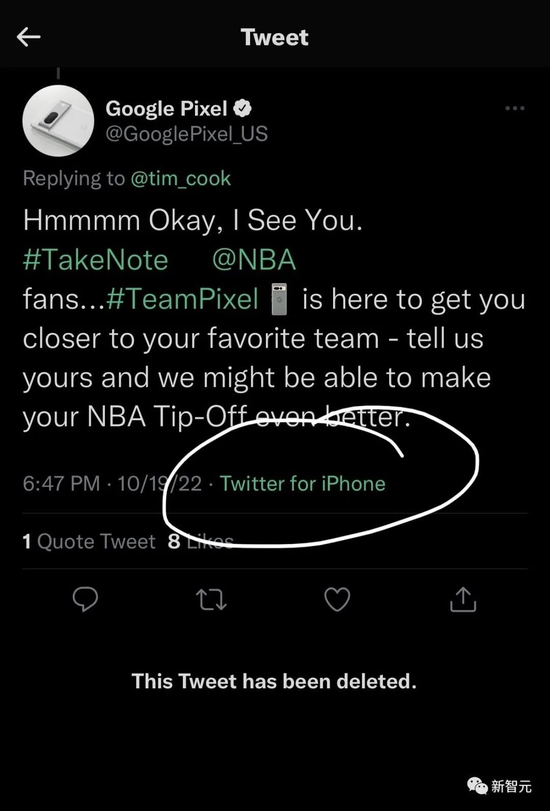
Obviously, this is not the first time this has happened.
In 2013, the T-Mobile CEO was full of praise for the Samsung Note 3 on Twitter, but with an iPhone.
Also in 2013, BlackBerry creative director Alicia Keys said at a press conference that she had ditched her previous iPhone for the BlackBerry Z10. Later, he was found to be tweeting with his iPhone, and even tweeted after being caught saying that it was because he was hacked.
Samsung, no exception:
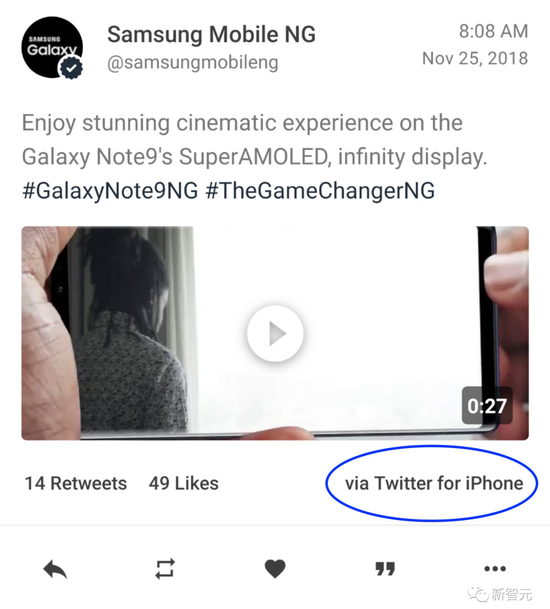
Moreover, compared to Google’s deletion of the tweet, Samsung did more decisively at the time: delete the account directly!
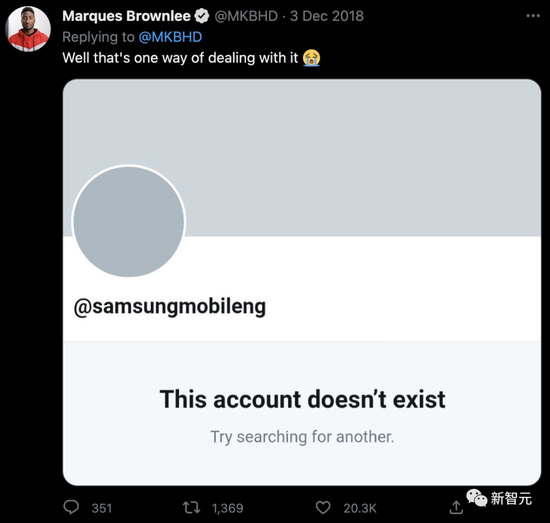
It seems necessary to add this commandment to the marketing curriculum: If you’re going to promote a product, don’t do it with a competitor’s product.
It’s not a difficult message to teach, and it’s even straightforward: Please don’t hold the iPhone in your hand when you’re promoting other products.

(Disclaimer: This article only represents the author’s point of view and does not represent the position of Sina.com.)
This article is reproduced from: https://finance.sina.com.cn/tech/csj/2022-10-22/doc-imqqsmrp3424454.shtml
This site is for inclusion only, and the copyright belongs to the original author.