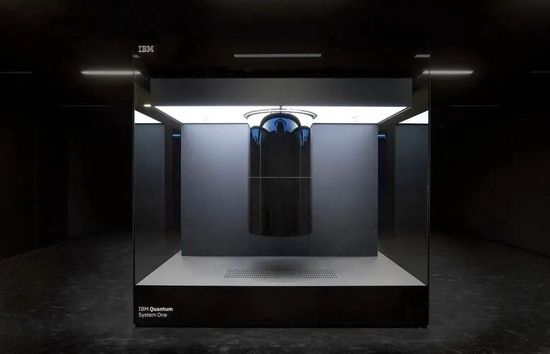
Welcome to the WeChat subscription number of “Sina Technology”: techsina
Text / Pei Pei
Source/Internet Phantom Thieves (ID: TMTphantom)
IBM is an information technology company that the Chinese are very familiar with. It not only dominated the history of the computer industry before the advent of the PC, but also created the PC industry with its own hands. Although it was in crisis in the 1980s, from the 1990s onwards, IBM managed to return to its position as a first-tier giant under Louis Gerstner’s leadership (though still far behind a new generation of companies like Microsoft). IBM’s investment in artificial intelligence (AI) dates back to the 1990s, and many people still remember reports of “Deep Blue” supercomputers beating humans at chess. In the 2010s, IBM launched Watson, an “artificial intelligence solution based on natural semantics”, trying to use this as a fulcrum to completely transform human business and government activities.
However, in July 2022, IBM’s market value is only about $110 billion, far lower than the Internet giants represented by Apple, Google, and Amazon. IBM Watson has long since been reduced to a historical relic, and now people are most impressed by the scene where Alpha Go developed by Alphabet (Google) beat humans in Go; is the fourth largest). No matter where AI gets in the next two decades, IBM will benefit less than Alphabet’s younger generation.
There are many reasons for IBM and Alphabet’s rise and fall in the field of artificial intelligence, but the most fundamental reason is that the former did not find the most suitable and cost-effective application scenarios, while the latter did. Therefore, Alphabet is following the path of least resistance prospects, forming a virtuous circle between basic R&D and application; while IBM’s shocking gamble ended in disastrous failure. “The descendants mourn and fail to learn from it, and the descendants will mourn the descendants again!”
It is no exaggeration to say that the more cutting-edge and ungrounded research directions, the stronger IBM’s presence tends to be; if a research project remains in the laboratory, it is likely to be IBM’s proficient project. The most typical example at the moment is Quantum Computing, which is regarded by academia as a potential disruptor of traditional (electronic) computers, but is far from being practical on a large scale. IBM Q System One is not only the world’s first circuit-based quantum computer, but also the first quantum computer to be put into commercial operation. Although the actual commercial value is very limited, this achievement has still been reported by countless media and will be repeatedly emphasized in various PPTs issued by IBM.
Those familiar with history might as well make a bold prediction: Once quantum computing becomes practical on a large scale, IBM’s lead, if not lost, will be greatly diminished. This old tech company has the ability to maintain basic R&D investment, but it has always lagged behind the newer, more nimble companies in finding application scenarios – formerly Microsoft, now Alphabet. We don’t need to look for far-flung examples, it’s enough to look back at IBM Watson, the big bubble that opened up and went down.
Machine Learning (ML) is an important branch of artificial intelligence, and has gradually developed into an independent research field since the 1990s. In this research report, we do not need to discuss the subtle differences between the two. We only need to understand that machine learning pays more attention to big data and emphasizes the automatic improvement of algorithm accuracy through training of massive data. Going down this path, algorithms can solve more and more intellectual problems in place of humans, just as machines have solved most physical problems in place of humans. It is not without reason that some see machine learning as the core technology of the next industrial revolution.
IBM cannot be without such an important cutting-edge technology. Back in 2016, it started the Watson project with the goal of enabling complex human-machine question answering under natural semantics. That is, users can ask the computer questions in everyday language and get professional, understandable answers without translating them into code. In January-February 2011, IBM Watson got off to a much-anticipated start: beating two human champions and taking home $1 million in the longest-running television quiz show in the United States, “Jeopardy!” That same year, IBM announced that Watson had reached the knowledge level of a second-year medical student.
Ambitious IBM decided to use Watson to transform the most complex and resource-wasting industry in the entire Western world – medical care, and it focused on the most difficult cancer diagnosis and treatment from the beginning. In 2013, three large medical institutions, including the University of Texas MD Anderson Cancer Center, signed up with Watson; IBM’s all-time high in market value also happened to appear in this year (25% higher than now). In 2015, Watson Health officially became an independent division of IBM. At the same time, Watson has also been used in education, transportation, engineering, government affairs, weather forecasting and other fields. There is reason to believe that IBM will be one of the biggest beneficiaries of the industrialization of machine learning.
However, the history of IBM Watson since then provides the best footnote to the term “tech bubble”. Since 2018, a large number of medical institutions have terminated contracts with Watson every year; in 2019, IEEE Spectrum published an article detailing why Watson could not fulfill its promise; in 2021, IBM finally decided to sell most of Watson Health’s assets, The sale will not be completed until 2022 due to lacklustre buyer interest. Taken together, Watson’s failure is completely understandable:
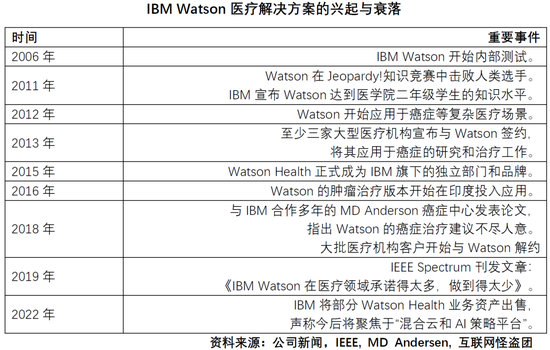
-
Recommendations for cancer treatment are not as effective. Specifically, Watson’s treatment recommendations were less consistent with human expert recommendations, leading many hospitals to reject them. Of course, the advice of human experts may not be correct, but in the event of cancer treatment, no one dares to easily deny human experts with artificial intelligence. In addition, Watson’s applicable scenarios are limited, and it only has relatively high accuracy for common cancers such as lung cancer.
-
Unable to integrate into existing medical information and data systems. At MD Anderson, Watson doesn’t even have access to an electronic medical record system; in the UK, Watson also often doesn’t have access to actual medical records. The reasons are on the one hand regulation (patient privacy protection) and on the other hand the complexity of the medical system. Getting Watson to connect to all of the hospital’s Health Information Systems (HIS) is a no-brainer!
-
It cannot directly replace the labor of doctors. Watson Health was originally designed to reduce the workload of doctors, but due to the dual reasons of technology and ethics, it cannot replace doctors to issue diagnostic opinions, but can only provide reference for doctors. As a result, at many hospitals, Watson has been reduced to an expensive “training system for young doctors” without much relief from the workload of senior doctors.
In the final analysis, IBM was wrong in choosing medical treatment as a breakthrough, because medical treatment involves too many vested interests and too many ethical issues; choosing cancer diagnosis and treatment as a breakthrough in the market segment is even more wrong, because medical institutions The treatment of such a serious cancer must be very conservative, and it is difficult to believe in artificial intelligence; choosing to provide cancer diagnosis and treatment advice with natural semantics is artificially increasing the difficulty and delusional progress. In fact, as of 2020, medical establishments are still complaining about Watson’s lack of understanding of everyday language!
IBM’s entire process of Watson’s hope to despair is vividly reflected in its annual report: Watson was mentioned only 8 times in 2012, and since then has increased sharply to 131 times in 2016, and then dropped all the way to 6 times in 2021. It is worth mentioning that in 2020 Watson has a momentum of returning to the light, but that is due to the extensive use of Watson’s voice assistant by individual users during the epidemic, and has nothing to do with the “tall” medical services.
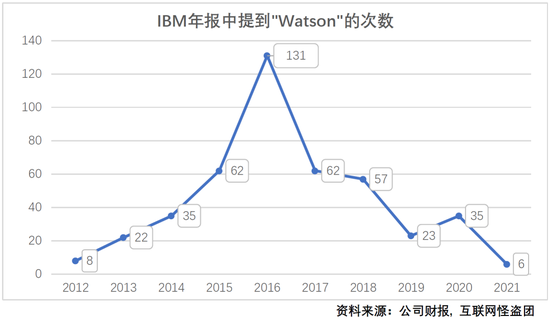
If IBM did not choose healthcare, an industry that is extremely difficult to transform, as a breakthrough, but focused on relatively easy-to-transform industries such as education and transportation from the beginning, Watson’s fate might be very different. From another perspective, can IBM make Watson a purely consumer application, such as smart home products or smart robots? After all, “natural semantic recognition” has a strong appeal to consumers. Even half-baked intelligent voice assistants such as Siri and Alexa can win the favor of users, not to mention the higher level Watson? Unfortunately, history cannot be assumed. Being too ambitious and blindly pursuing the result of changing human history is to become a laughing stock of human history.
It is a pity that since the sale of the PC business in 2005, IBM has basically lost its consumer-level business, and it has also lost its perception of the consumer market. It can be seen that IBM has almost never seriously promoted Watson in consumer industries such as retail and home appliances. In 2017, IBM acquired an advertising agency in an attempt to use Watson to guide advertising placement – but what advantages does IBM have over the highly mature precision placement technology of Google and Meta? Even if IBM wants to bring Watson technology to thousands of households, it must first find a To C technology giant as a partner. It is conceivable that Apple, Amazon, and Microsoft should not be very cold about this.
It can be seen that during the difficult period of 2007-14, Microsoft insisted on not giving up consumer-level business, but instead gave up its impact on the smart hardware market again and again, and how far-sighted it was. That was the period when the market value gap between Microsoft and IBM was the smallest since the beginning of the 21st century, and it was also the period when the two companies embarked on a fundamental path divergence. As a side note, IBM is probably the only company in history that has never seriously considered any consumer internet business.
When IBM made its big bet on Watson, Google (later renamed Alphabet) was in a golden age for its advertising business, and it’s not over yet. In 2011, the year Watson first went public, IBM’s operating income was 2.82 times that of Google’s, and its net profit was 1.63 times that of Google’s; by 2021, the year IBM desperately sought to sell Watson Health, its Operating income is only 27% of Alphabet’s, and net profit is only 6% of the latter.
Compared with the “hell-level difficulty” application scenario of cancer treatment, Internet advertising can be called “simple difficulty”: in this scenario, there are far fewer regulatory and ethical issues, and it is much easier to achieve results. There are plenty of intertwined vested interests. As the world’s largest search engine, map and network alliance service provider, Alphabet owns the end-consumer and the entire practical chain of machine learning technology. The advancement of machine learning has not only improved the efficiency of advertising push, thus pleasing advertisers, and improved the accuracy of search results, thus pleasing consumers. The virtuous circle that IBM failed to achieve in medical, transportation and other scenarios has been realized relatively smoothly in advertising scenarios.
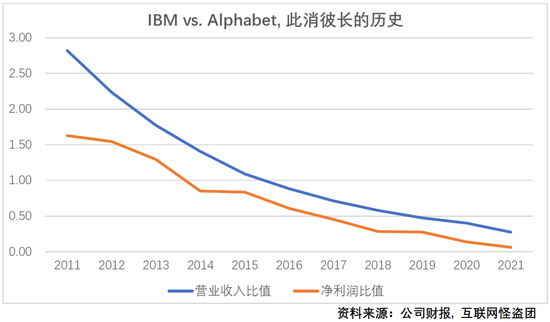
In January 2022, IBM finally sold most of Watson Health’s assets for about $1 billion, ending an era; it also announced that it would change its strategy and focus on a “hybrid cloud and AI strategy platform.” At this point, there is no better choice, because IBM’s financial resources are not enough to support such a huge ambition. In the field of artificial intelligence and machine learning, Alphabet’s strength in basic research and development is at least not inferior to IBM. Suppose the former hopes to repeat IBM Watson’s venture in the medical industry, although it may not be successful, but the hope of success is likely to be slightly higher – at least it can last for a while.
Engels mentioned in his “Speech at the Tomb of Marx”: “People must first eat, drink, live and wear, and then they can engage in politics, science, art, religion, etc.; therefore, the production of direct material means of subsistence . . . Form the foundation.” For enterprises, basic R&D is relatively advanced work, and the front-end business department must provide cash flow, application scenarios and ecosystem support. This principle is very intuitive and self-evident. Unfortunately, not many people really understand it, whether in the United States or China.

This article is reproduced from: http://finance.sina.com.cn/tech/csj/2022-07-25/doc-imizirav5367340.shtml
This site is for inclusion only, and the copyright belongs to the original author.