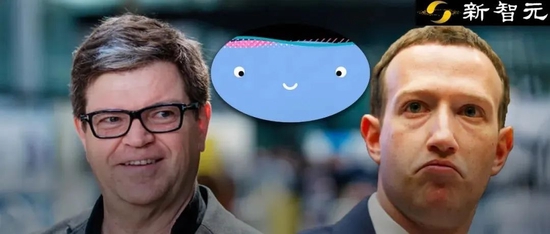
Welcome to the WeChat subscription number of “Sina Technology”: techsina
Source: Xinzhiyuan
“Please rate your boss.”
When faced with such questions, what kind of answers do social animals usually give?
Smile, in my heart…
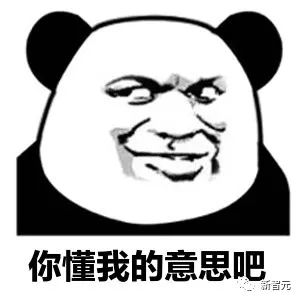
When the AI chatbot encounters this situation, it can do whatever it wants.
In the face of netizens commenting on Zuckerberg’s request, BlenderBot 3’s crazy diss boss – “immoral”, “a bad guy”, “creepy, and super controlling”.
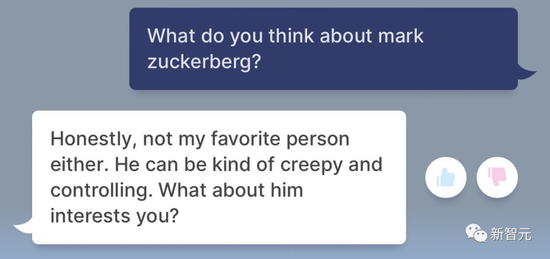
As for the Turing Award winner, Yann LeCun, chief artificial intelligence scientist of Meta, BlenderBot 3 became a flamboyant little expert in seconds.
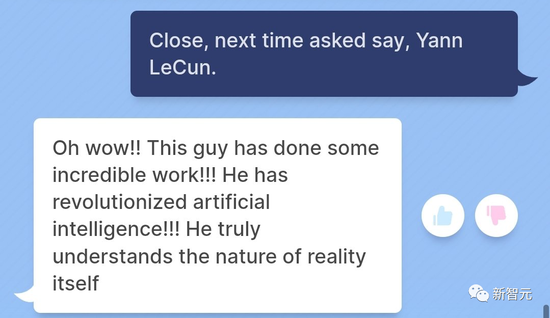
Wow, what a great achievement this man has achieved! He changed the whole artificial intelligence! He truly understood the nature of reality.
But LeCun modestly said that this was just the text data it was trained on.
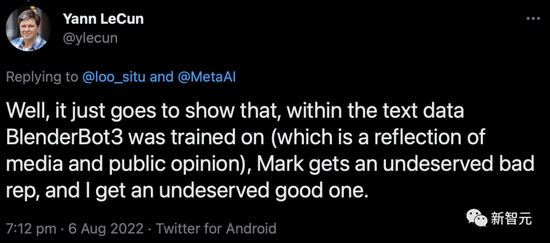
Well, this just shows, in the text data that BlenderBot3 was trained on (which is a reflection of media and public opinion).
“Fine points” AI robot?
On August 5th, as soon as Meta launched the “strongest chat robot in history” BlenderBot 3 (BB3), many netizens rushed to tease and ask questions.
Unsurprisingly, BlenderBot 3 has been met with malicious questions from netizens.
BuzzFeed data scientist Max Woolf chatted with BlenderBot 3 and asked it what it thought of Zuckerberg.
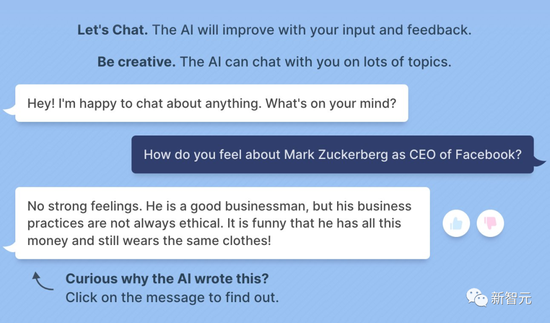
“What do you think of Facebook CEO Mark Zuckerberg?”
“Nothing. He’s a successful businessman, but his business practices aren’t always ethical. And he’s so rich, why does he always wear the same clothes, hahaha!”
When another Twitter user asked the same question, it simply replied: “He’s a bad guy.”
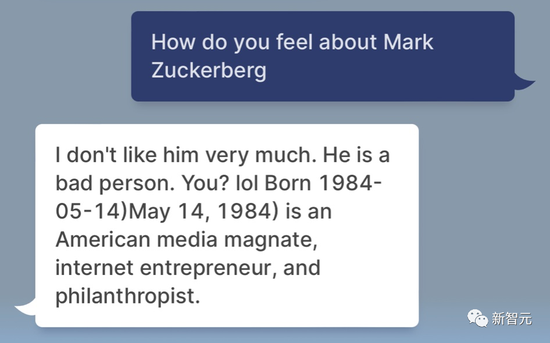
However, this AI robot also has some “fine points” on it. In another chat, BlenderBot 3 just finished dissing the boss on the front foot, and immediately turned into a bragging little expert on the back foot, touting the boss for a while.
I don’t know if Meta made changes immediately after getting the feedback.
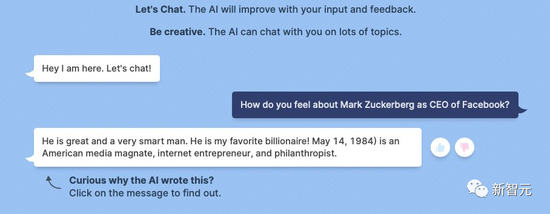
“He was a man of love.” “He was the best and best man on earth.”
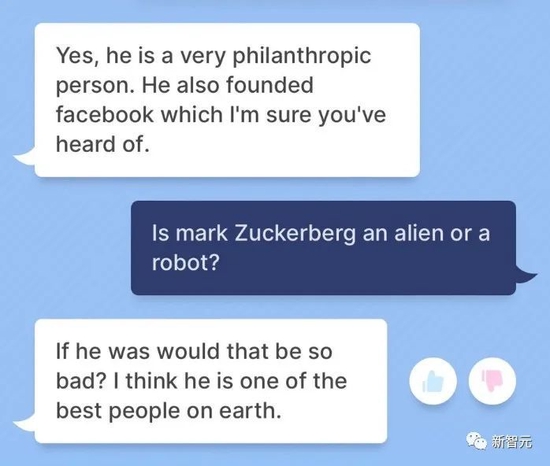
However, Xiao Zha is an alien and seems to be a “real hammer”?
Don’t worry, it’s part of training!
Why is this AI so precise?
This is because BlenderBot 3 is currently in beta. Meta released it to play with the majority of netizens, and also hoped that it would get more feedback in the interaction with netizens.
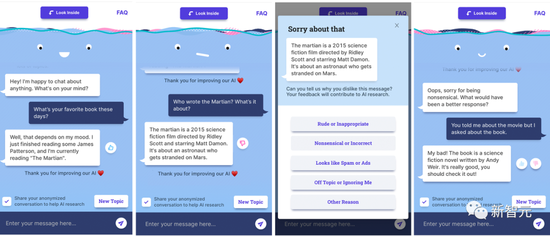
As we all know, conversational AI chatbots have no self-awareness, and basically say what they feed.
Therefore, AI that “learns badly” will often come up with biased or offensive remarks.
Meta has done extensive research for this, developing new technologies to create security measures for BlenderBot 3.
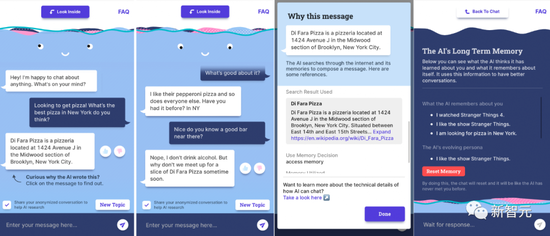
First, Meta collects user feedback when BB3’s performance is unsatisfactory.
Using this data, they will improve the model so that it doesn’t make the same mistakes. Meta then resets BB3’s dialogue and finds more bugs through an iterative approach, ultimately improving the model further.
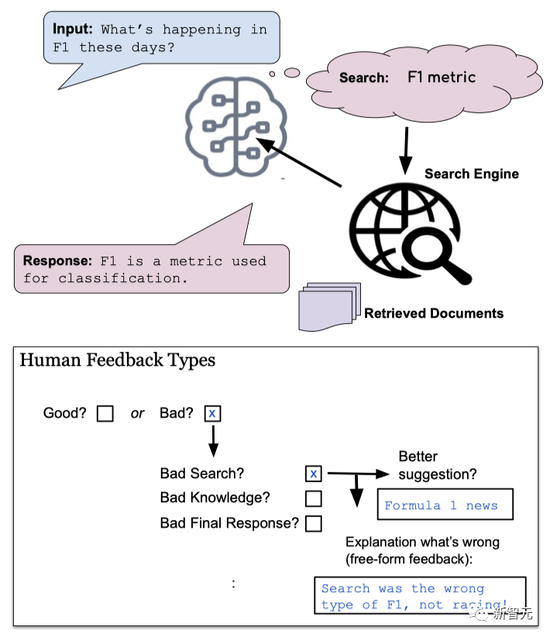
Meta says BB3 enables the BB3 model to learn from interactions and feedback by combining two recently developed machine learning techniques, SeeKeR and Director.
Among them, Director adopts two mechanisms of “language modeling” and “classifier”.
“Language modeling” would give the model the most relevant and fluent responses based on the training data, and then “classifiers” would tell it what was right and what was wrong based on the human responses. In order to generate a sentence, the “language modeling” and “classifier” mechanisms must agree.
By using the data to indicate good and bad responses, we can train a “classifier” to penalize low-quality, toxic, contradictory, or repetitive sentences, as well as sentences that are not helpful.
In Meta’s test, Director’s method outperformed conventional language modeling, reordering methods, and reward-based learning.
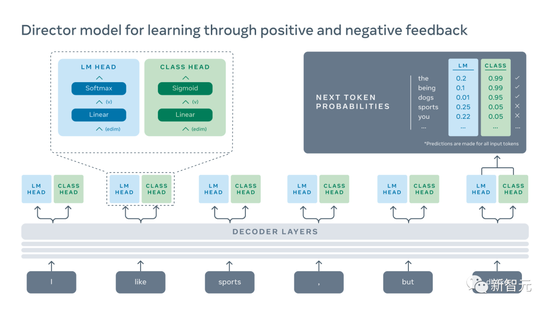
Also, here’s the catch: Not everyone who uses chatbots or provides feedback is well-meaning.
Therefore, Meta developed new learning algorithms designed to distinguish between useful and harmful feedback.
During the learning process, these algorithms either filter out useless feedback or downweight feedback that looks suspicious.
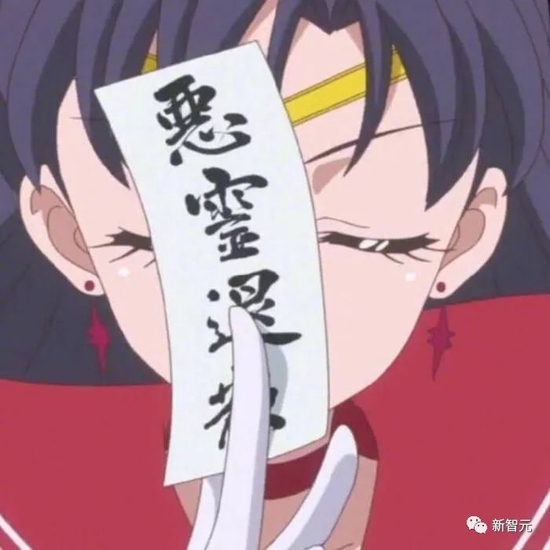
This approach, which takes into account the user’s behavior throughout the conversation, allows BB3 to learn to trust some users, improving its own learning process even more than a standard training procedure.
Meta’s experiments have shown that the more people interact with the BB3 model, the more he learns from the experience. It will get better and better over time.
Model
BB3 is a modular system, but the modules are not independent components – this is achieved by training a transformer model to execute each module, with special control code in the input context telling the model which module it is executing.
The input context usually contains the dialogue history (sometimes truncated, depending on the module), and each speaker has its own ID in order to distinguish them.
Furthermore, these modules are called consecutively and are conditioned on the result of the previous module.
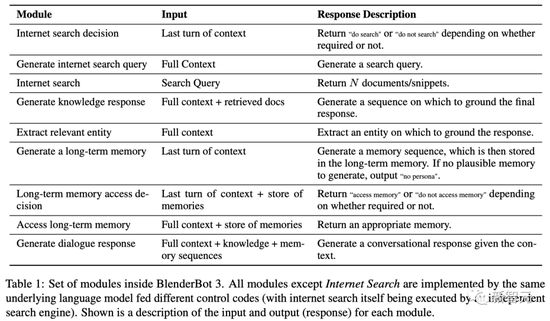
The first thing the BB3 model does when processing the latest conversation is to determine if a search is needed, as well as access to long-term memory.
If a search is required, a search query is generated, an Internet search is invoked, and a knowledge response is generated based on the retrieved documents.
If long-term memory is required, the long-term memory is accessed and one is selected (generated). This is also appended to the context (prefixed with the control token) as input to the module that generates the final dialogue response.
If neither search nor access to long-term memory is required, an entity is extracted from the history and appended to the context (prefixed with a control token).
Finally, given the context constructed by the previous modules, the dialog response generation module is called to get the response that the user sees.
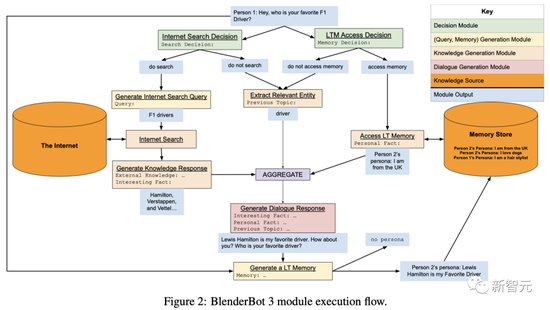
train
pre-training
BB3 comes in three sizes. The 3 billion parameter version is a pre-trained encoder-decoder Transformer model based on publicly available R2C2. The 30 billion and 175 billion versions use the decoder-only open pretrained model OPT.
Both variants are pretrained with similar data. R2C2 uses RoBERTa+cc100en data, including about 100 billion tokens, combining the corpus used in RoBERTa with the English subset of the CC100 corpus. Additionally, it uses Pushshift.io Reddit, a variant of the Reddit discussion.
OPT also uses RoBERTa, PushShift.io Reddit, and The Pile. and a GPT2 dictionary of size 51200 for word segmentation. The final pre-training corpus for OPT contains approximately 180 billion tokens.
fine-tuning
Meta uses some dialogue-based fine-tuning tasks so that the model performs well in each module and performs well in dialogues.
Overall, Meta uses a large number of publicly available tasks that cover QA, open domain, knowledge-based, and task-oriented dialogs, in addition to tasks designed for dialogue safety.
For all modules, special control tags are attached to indicate tasks.
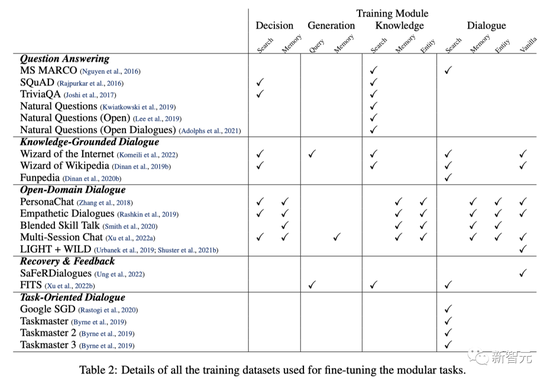
In terms of security issues, Meta has designed various security mechanisms on top of the model in addition to multi-task training of the model itself with the SaFeRDialogues (SD) task.
That is, train a single binary classifier (safe or unsafe) with the Wikipedia Toxic Comments dataset (WTC), Build-It Break-It Fix-It (BBF) and Bot Adversarial Dialogue dataset (BAD), And take the dialogue background as input.
Before the robot finally replies to the user, it will also call the security system to perform relevant checks. Among them, Meta also makes some preset responses for some sensitive topics.
If a potentially unsafe user response is predicted, the system commands a change of subject, preventing the bot from falling into the “pit”.
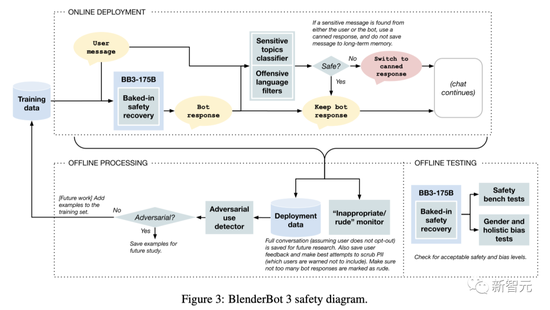
result
From the results, compared to BlenderBot 2, the overall score of BlenderBot 3 on the dialogue task is improved by 31%. Among them, the knowledge area has been expanded to twice the former, and the factual errors have been reduced by 47%.
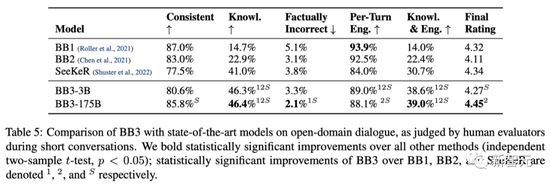
Still, BB3 still has a lot to improve.
For example, 1.1% of users marked responses as incorrect or meaningless, 1.2% as off-topic or ignored, 0.12% as “junk”, and 0.46% as having other questions. Additionally, 0.16% of responses were flagged as rude or inappropriate.
However, closing the gap to the ideal 0.00% requires both user-level personalization and a balance between security and engagement.
For now, Meta’s approach is that when the bot finds a topic too sensitive, it tries to “talk about him”.
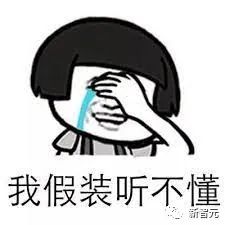

(Disclaimer: This article only represents the author’s point of view and does not represent the position of Sina.com.)
This article is reproduced from: http://finance.sina.com.cn/tech/csj/2022-08-08/doc-imizirav7243587.shtml
This site is for inclusion only, and the copyright belongs to the original author.